Gender bias in search algorithms has effect on users, new study finds
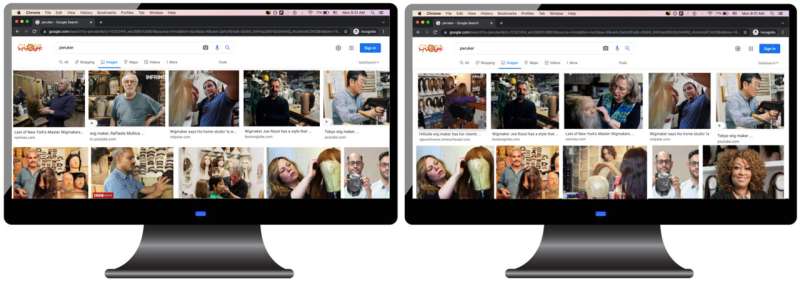
Gender-neutral internet searches yield results that nonetheless produce male-dominated output, finds a new study by a team of psychology researchers. Moreover, these search results have an effect on users by promoting gender bias and potentially influencing hiring decisions.
The work, which appears in the journal Proceedings of the National Academy of Sciences (PNAS), is among the latest to uncover how artificial intelligence (AI) can alter our perceptions and actions.
"There is increasing concern that algorithms used by modern AI systems produce discriminatory outputs, presumably because they are trained on data in which societal biases are embedded," says Madalina Vlasceanu, a postdoctoral fellow in New York University's Department of Psychology and the paper's lead author. "As a consequence, their use by humans may result in the propagation, rather than reduction, of existing disparities."
"These findings call for a model of ethical AI that combines human psychology with computational and sociological approaches to illuminate the formation, operation, and mitigation of algorithmic bias," adds author David Amodio, a professor in NYU's Department of Psychology and the University of Amsterdam.
Technology experts have expressed concern that algorithms used by modern AI systems produce discriminatory outputs, presumably because they are trained on data in which societal biases are ingrained.
"Certain 1950s ideas about gender are actually still embedded in our database systems," Meredith Broussard, author of "Artificial Unintelligence: How Computers Misunderstand the World" and a professor at NYU's Arthur L. Carter Journalism Institute, told the Markup earlier this year.
The use of AI by human decision makers may result in the propagation, rather than reduction, of existing disparities, Vlasceanu and Amodio say.
To address this possibility, they conducted studies that sought to determine whether the degree of inequality within a society relates to patterns of bias in algorithmic output and, if so, whether exposure to such output could influence human decision makers to act in accordance with these biases.
First, they drew from the Global Gender Gap Index (GGGI), which contains rankings of gender inequality for more than 150 countries. The GGGI represents the magnitude of gender inequality in economic participation and opportunity, educational attainment, health and survival, and political empowerment in 153 nations, thereby providing societal-level gender inequality scores for each country.
Next, to assess possible gender bias in search results, or algorithmic output, they examined whether words that should refer with equal probability to a man or a woman, such as "person," "student," or "human," are more often assumed to be a man. Here, they conducted Google image searches for "person" within a nation (in its dominant local language) across 37 countries. The results showed that the proportion of male images yielded from these searches was higher in nations with greater gender inequality, revealing that algorithmic gender bias tracks with societal gender inequality.
The researchers repeated the study three months later with a sample of 52 countries, including 31 from the first study. The results were consistent with those from the initial study, reaffirming societal-level gender disparities are reflected in algorithmic output (i.e. internet searches).
Vlasceanu and Amodio then sought to determine whether exposure to such algorithmic outputs—search-engine results—can shape people's perceptions and decisions in ways consistent with pre-existing societal inequalities.
To do so, they conducted a series of experiments involving a total of nearly 400 female and male U.S. participants.
In these experiments, the participants were told they were viewing Google image search results of four professions they were likely to be unfamiliar with: chandler, draper, peruker, and lapidary. The gender composition of each profession's image set was selected to represent the Google image search results for the keyword "person" for nations with high global gender inequality scores (roughly 90% men to 10% women in Hungary or Turkey) as well as those with low global gender inequality scores (roughly 50% men to 50% women in Iceland or Finland) from the 52-nation study above. This allowed the researchers to mimic the results of internet searches in different countries.
Prior to viewing the search results, the participants provided prototypicality judgements regarding each profession (e.g., "Who is more likely to be a peruker, a man or a woman?"), which served as a baseline assessment of their perceptions. Here, the participants, both female and male, judged members of these professions as more likely to be a man than a woman.
However, when asked these same questions after viewing the image search results, the participants in the low-inequality conditions reversed their male-biased prototypes relative to the baseline assessment. By contrast, those in the high-inequality condition maintained their male-biased perceptions, thereby reinforcing their perceptions of these prototypes.
The researchers then assessed how biases driven by internet searches could potentially influence hiring decisions. To do so, they asked participants to judge the likelihood that a man or woman would be hired in each profession ("What type of person is most likely to be hired as a peruker?") and, when presented with images of two job candidates (one woman and one man) for a position in that profession, to make their own hiring choice (e.g., "Choose one of these applicants for a job as a peruker.").
Consistent with the other experimental results, exposure to images in the low-inequality condition produced more egalitarian judgments of male vs. female hiring tendencies within a profession and a higher likelihood of choosing a woman job candidate compared with exposure to image sets in the high-inequality condition.
"These results suggest a cycle of bias propagation between society, AI, and users," Vlasceanu and Amodio write, adding that the "findings demonstrate that societal levels of inequality are evident in internet search algorithms and that exposure to this algorithmic output can lead human users to think and potentially act in ways that reinforce the societal inequality."
More information: Madalina Vlasceanu et al, Propagation of societal gender inequality by internet search algorithms, Proceedings of the National Academy of Sciences (2022). DOI: 10.1073/pnas.2204529119
Journal information: Proceedings of the National Academy of Sciences
Provided by New York University